Suncorp has built a generative AI engine it is calling 'SunGPT' to improve insurance agents’ access to information.
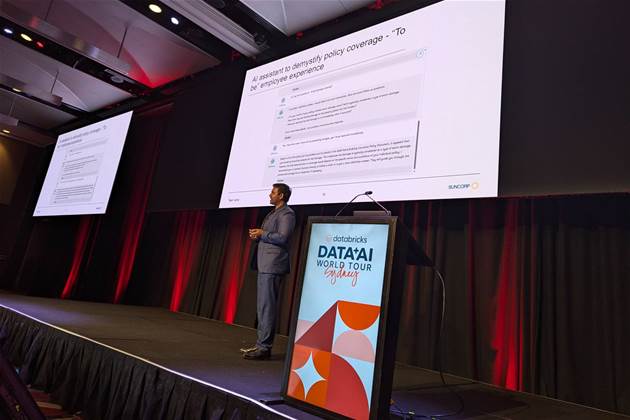
The engine uses Databricks’ Mosaic AI services to host and manage multiple large language models (LLMs) that agents can use to answer customer questions relating to onboarding, claims and policies.
This includes Mosaic AI Model Serving, a unified service for deploying, governing and querying AI models, to host and manage LLMs spanning ChatGPT, Azure Open and AWS Bedrock.
Suncorp is currently focusing SunGPT on use cases such as personalised customer service, cyber security and operational efficiency, compliance and risk management, and market research and strategy.
In addition, Suncorp also has another 20 use cases in development, with the goal of integrating them into the insurer’s business processes this year.
Priyanka Paranagama, Suncorp's CTO and EGM of AI Transformation, told iTnews that SunGPT "is Suncorp's AI platform that utilises Databricks and connects our customer and operational data with the best generative AI models out there, in a safe and secure manner."
"It is a platform Suncorp's data scientists are already using to build apps to help our employees in specific use cases," Paranagama said.
"One example is 'single view of claim, that has been deployed on this platform and is now being used by our home insurance claims team and is scaling up to support more than 1000 employees."
'Single view of claim' was referenced at the insurer's recent FY24 results, though it did not specify at the time how it was delivering this capability.
Speaking at Databricks’ Data and AI Summit in Sydney, Suncorp AI practice executive Kranthi Nekkalapu described SunGPT as a “combination of frameworks, agentic workflows, a lot of code we have built, guardrails and access to different models through secured connections”.
The models draw on both unstructured information and data to deliver answers to agents’ questions.
The orchestration layer, Nekkalapu added, is able to get data from different places in real-time.
The right balance
Nekkalapu said the SunGPT build encountered challenges around accuracy, scalability, performance and cost.
Speaking about perfecting the engine’s accuracy, Nekkalapu said: “There are lots of other scenarios where you think the model is nearly there, but the information is not sufficient.”
Meanwhile, scalability and performance were more about SunGPT’s ability to serve thousands of people in real-time.
“You don’t want [the agents] to ask the question and then have to wait a minute or two for answers,” Nekkalapu said. “Matching those expectations is important – as is cost.”
“One thing with generative AI, as opposed to traditional machine learning, is cost,” he continued. “You realise that when you get these models; when you deploy them, you need GPUs, and GPUs are so expensive. And costs are going to blow up, even before we realise it.
“You have to optimise all of these together. If you try to reduce costs, accuracy will take a hit. If you want a very accurate solution, and you are ok with [the] cost, that’s not going to return a response in seconds.”
According to Nekkalapu, a considerable amount of time was dedicated to "finding the right balance between these four".
Managing risk
The process of developing SunGPT required considerable testing and feedback due to the potential risks of using generative AI content.
“All these generative AI applications have their own risk,” Nekkalapu said. “We are generating text so there is always a chance you might generate something that doesn’t align with your principles.”
Using Databricks Lakehouse Monitoring proved to be "very important” for monitoring SunGPT constantly.
In addition, Suncorp sought assistance from subject matter experts (SMEs), who were requested to provide feedback on both the prototype’s accuracy and user experience.
“We involved our SMEs very early in the process because again, as opposed to machine learning, you don’t have a clear, objective way of evaluation. What might be a good response to a technical expert may seem a completely wrong response when reviewed by a subject matter expert," he said.