NAB has initiated the first of what is likely to become an annual refresh of its data strategy, a shade over a year since unveiling its first data foray into the cloud.
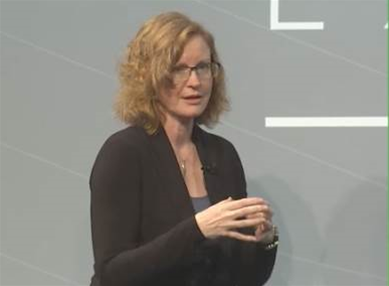
Chief data officer Glenda Crisp revealed the bank’s expanded data architecture - the product of the first year of work - at the recent AWS Summit in Sydney.
At an executive forum run in parallel to the summit, Crisp separately revealed the data strategy is now up for review, as well as some of the metrics NAB is using to account for its efforts.
“I do personally believe in refreshing the data strategy on an annual basis, so we're about to kick off the refresh,” she told the May event.
“It won't be as big of a rewrite this year as it was last year, but I think it's important to reflect what are the changes that have happened in the market, as well as what's the progress that we've made since we started last year.”
Crisp said the initial rewrite of the bank’s data strategy, coinciding with her appointment last year, was a joint effort by “a bunch of different NAB people”.
“We put it through a formal challenge process internally with senior executives to make sure we weren't missing anything,” she said.
The collaborative approach to its development was seen as new in some parts of the bank.
“I have had people tell me that the fact that I actually put it out for challenge and debate was a new thing, and that I factored in their feedback was [also] a new thing,” Crisp said.
“I think especially the finance team and the risk team really liked that I had metrics. And certainly the board was very happy to see that we had specific metrics and targets that we wanted to achieve.”
Crisp said that the metrics around the data strategy focused on measuring both progress and delivered value.
“You have to measure the progress that you're making against the strategy, and are you achieving the outcomes you set out to get but also the value to the business,” she said.
“You also need to balance leading and lagging indicators. So, for example, around the [data] lake that we're building, the lagging indicator - the value metric - is the speed at which we can provide data to analytics teams.
“We benchmarked it against our legacy environment and then we set targets for the next three years, and we have a plan as to how we're going to land our data.
“The leading indicator on this one is the number of sources that we've landed in the lake. So if I don't get the sources landed, then I know I'm not going to achieve that value metric that my business partners need.
Further metrics targeted cost savings and data quality experienced by internal users.
Crisp suggested further metrics had been wrapped around complaints relating to data quality issues.
“I've been working with the team to try to benchmark and baseline the number of customer complaints related to data issues, because that's something that I want to track super closely, because I believe the right number is zero,” Crisp said.
“There should be no complaints about data, so we're just baselining that right now so that then we can start to drive improvements.”
Crisp said the process of landing different sources of data into the data lake - which now goes by the name of NAB data hub or NDH - is being kept a relatively fluid affair.
She said her team took “first stab” at prioritising which data to land in the lake.
“We did a lot of analysis on which sources are most often used,” Crisp said.
“[From that] we created a list with tranches and then I have an analytics committee [with] representatives from across the bank, and I put it in front of them and said, 'OK, here's the straw model, tell me what's wrong and tell me where I should re-prioritise'.
“That resulted in us rejigging the list as to what landed first, second, third. We've kept it relatively fluid, so we lock it down a quarter at a time - and I still have my next set of three quarters out for what I want to land.
“But constantly, every time somebody says, 'Hey, I think this data is really important, and I've got a use case for it’, ‘I've got some really great analytics thing I need to do’, or ‘I have regulatory requirement I need to address', then we'll just re-prioritise.
“So as long as you can make a case, give me an argument as to the value that it's going to generate for the bank, then I'm happy to move the data source up the list.
“That's really worked quite well for us.”
Crisp remained relatively quiet on data use cases aside from those already out in the public domain, though she suggested considerable efforts were being invested in initiatives targeting fraud.
“The advanced analytics team have been able to do some really interesting work around machine learning, because we started building our advanced analytics platform at the same time as the lake,” she said.
“We've been able to do some interesting things around fraud and I don't like to talk about this too much.”